LLM judges are the de facto standard for evaluating anything related to LLMs. Human evaluations are too expensive and difficult to scale, so LLMs are a practical alternative.
But judges aren’t perfect. Here, we will examine some of the common problems with LLM judges and explore different ways to deal with them.
Please note that this blog will not cover non-LLM evaluations!
Let us first review what Agent Evaluation looks like in Databricks.
LLM-as-a-judge is a common evaluation technique; instead of using a human to evaluate a text response, we use an LLM. Mosaic AI Agent Evaluation allows you to systematically assess the quality of your agentic applications. This includes the use of LLM judges.
There are several built-in judges that can be used, including:
Correctness judge: assesses whether response is accurate
Helpfulness judge: assesses if response satisfies the user
Harmlessness judge: assesses if response avoids harmful content
Coherence judge: assesses if response is logical
Relevance judge: assesses whether response addresses the query
Given an evaluation set, you can use these judges to evaluate. Each judge takes a different set of inputs; for example, the Correctness judge requires a request, a response, and an expected response, but the Harmlessness judge only requires a request and a response. You can take a look at what judges are available and how to use them here.
LLMs have a hard time with numbers.
Let me show you what a basic implementation looks like. Using the databricks callable judge SDK, you can use the correctness judge like:
from databricks.agents.evals import judges
assessment = judges.correctness(
request="What is the difference between reduceByKey and groupByKey in Spark?",
response="reduceByKey aggregates data before shuffling, whereas groupByKey shuffles all data, making reduceByKey more efficient.",
expected_facts=[
"reduceByKey aggregates data before shuffling",
"groupByKey shuffles all data",
]
)
We can see that an assessment contains information something like this:
Assessment:
error_code=None
error_message=None
metadata={}
name='correctness'
rationale="..."
value=CategoricalRating.YES
The value that the assessment returned is categorical. LLMs notably struggle quite a lot with numerical scoring. Some studies show that they have preferences for certain values. Other studies often show them clustering around the highest and lowest values, instead of utilizing the full range.
Let us assume you have already created a judge to output scores from 1 to 10. When graphing the scores with the “ideal” scores, you could see something like this:
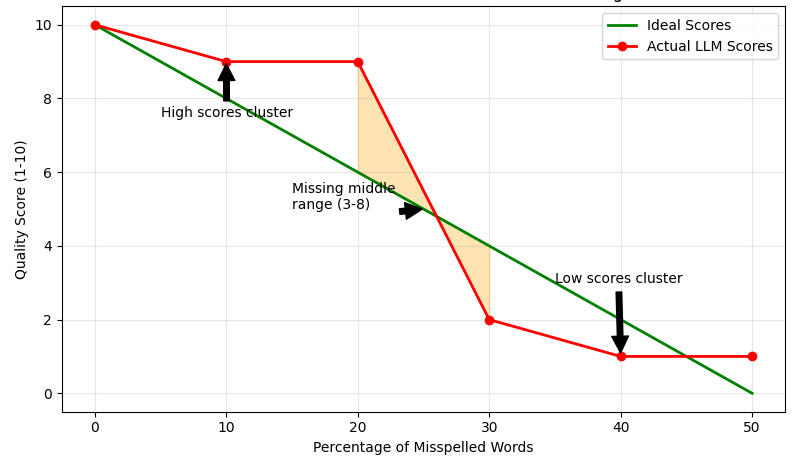
The built-in judge already includes a categorical value instead of a numerical one, but if you need numerical ratings, prompt the judge with an explanation for each of the scores. In MLFlow, you can include evaluation examples when defining different evaluation metrics.
average_example = EvaluationExample(
input="What are the main types of horse breeds?",
output="The main horse breeds include Arabian, Thoroughbred, Quarter Horse, Appaloosa, Morgan, Tennessee Walker, Clydesdale, and Mustang. Arabians are known for endurance, Thoroughbreds for racing, Quarter Horses for sprinting, and Clydesdales for their size and strength.",
score=5,
justification="This response correctly lists 8 common horse breeds and provides brief descriptions for 4 of them, but the descriptions are very basic and only cover half of the breeds mentioned. It lacks depth about breed characteristics, historical origins, or typical uses.",
grading_context={
"targets": "There are numerous horse breeds worldwide, with common breeds including Arabian, Thoroughbred, Quarter Horse, Appaloosa, Morgan, Tennessee Walker, Andalusian, Friesian, Clydesdale, Percheron, Mustang, and Shetland Pony. Each breed has distinctive physical traits, temperaments, and was developed for specific purposes like racing, work, or riding."
},
)
horse_breed_similarity_metric = answer_similarity(
examples=[poor_example, average_example, excellent_example])
LLMs like long answers.
In my last example regarding horses, you can see that the average example was scored lower than it would have been because it lacked ‘depth.’ Unfortunately, lots of LLMs equate depth with a lot of unnecessary chatter. When graphing scores of responses of equal quality, you may see long responses rewarded more than short responses, like:
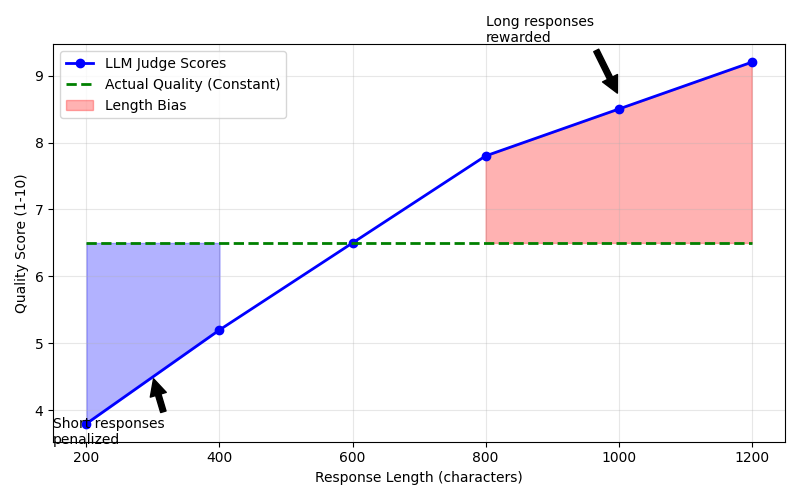
LLM judges tend to prefer longer outputs. This makes sense if you have ever used one of these chat bots. Depending on your use case, this might not be preferable. When I am talking to a customer service chatbot, for example, I get frustrated when it responds with paragraph long responses to my simple questions. Conciseness is incredibly important. This problem could also mean that more accurate responses are drowned out by rambling, semi-accurate responses. Observe what is getting approved by your judge to make sure the LLM is not avoiding brevity.
If you are noticing that only long answers are getting approved, you can instead simply adjust scores based on the length, penalizing answers that are ‘too’ long.
Let us assume that you have extracted the base score from the judge. You can have a simple function that penalizes a longer answer, if it surpasses a hardcoded threshold.
length_ratio = response_length / max(1, request_length)
def linear_verbosity_adjustment(length_ratio, base_score):
threshold = 3.0
if length_ratio <= threshold:
return 0
else:
return min(base_score * 0.3, (length_ratio - threshold) * 0.5)
But you can also approach this in a more sophisticated manner. In this paper, they fit a regression model to predict “what would the score be if the responses all had the same length?” This improved correlation with human preferences from 0.94 to 0.98, but in most cases, however, this is overkill.
LLMs are biased towards themselves.
We have also seen that LLM judges have a preference for text with lower perplexity. This suggests that LLMs prefer language similar to language they were trained on. This can lead to your evaluators assigning higher scores to outputs generated by their own kind. For example, you can no longer trust a GPT model to evaluate a Llama 8B model against a GPT 4o-mini model without bias.
You can mitigate this bias by using a jury-- a collection of LLM judges instead. The goal here is to use LLMs from different families, so one LLM’s bias towards the answer does not prevent you from understanding the quality of the response. You can create a custom metric in Databricks to do this. Here, I am defining three different judges using different LLMs with the same prompt.
import mlflow
from mlflow.metrics.genai import make_genai_metric_from_prompt
from databricks.agents.evals import metric
from databricks.agents.evals import judges
from mlflow.evaluation import Assessment
judge_prompt = """
Determine if this response accurately covers all expected facts.
Request: '{inputs}'
Response: '{response}'
"""
llama_judge = make_genai_metric_from_prompt(
name="accuracy_judge1",
judge_prompt=judge_prompt,
model="endpoints:/databricks-meta-llama-3-1-405b-instruct",
metric_metadata={"assessment_type": "ANSWER"}
)
claude_judge = make_genai_metric_from_prompt(
name="accuracy_judge2",
judge_prompt=judge_prompt,
model="endpoints:/databricks-claude-3-7-sonnet",
metric_metadata={"assessment_type": "ANSWER"},
)
gpt_judge = make_genai_metric_from_prompt(
name="accuracy_judge3",
judge_prompt=judge_prompt,
model="endpoints:/test-gpt-endpoint",
metric_metadata={"assessment_type": "ANSWER"},
)
Then, take all of these individual judges and define a custom metric. I am averaging the scores outputted from each judge here, but if you want to avoid numeric values, you can instead average across boolean values. If you are seeing that the metric is too ‘easy’ to pass, you can set a numeric threshold that must be passed by each judge. This would make sense for higher risk use cases.
@metric
def llm_jury(request, response):
inputs = request['messages'][0]['content']
llama_metric_result = llama_judge(inputs=inputs, response=response)
claude_metric_result = claude_judge(inputs=inputs, response=response)
gpt_metric_result = gpt_judge(inputs=inputs, response=response)
int_score = llama_metric_result.scores[0] + claude_metric_result.scores[0] + gpt_metric_result.scores[0]
int_score = int_score/3
return [
Assessment(
name="llm_jury_score",
value=int_score,
rationale=f"LLAMA: {llama_metric_result.scores[0]:.2f}, Claude: {claude_metric_result.scores[0]:.2f}, GPT: {gpt_metric_result.scores[0]:.2f}"
)
]
LLMs produce inconsistent evals.
Judges can output significantly different results if you prompt it multiple times. By default, using make_genai_metric_from_prompt
uses temperature=0.0
and top_p=1.0
, but there is still a chance that the LLM judges output different results (if you have time, read Austin’s blog on LLM non-determinism here).
You can test consistency with a judge by prompting it multiple times. In a perfect world, the same prompt would get the same scores, but other times, you may get something like this:
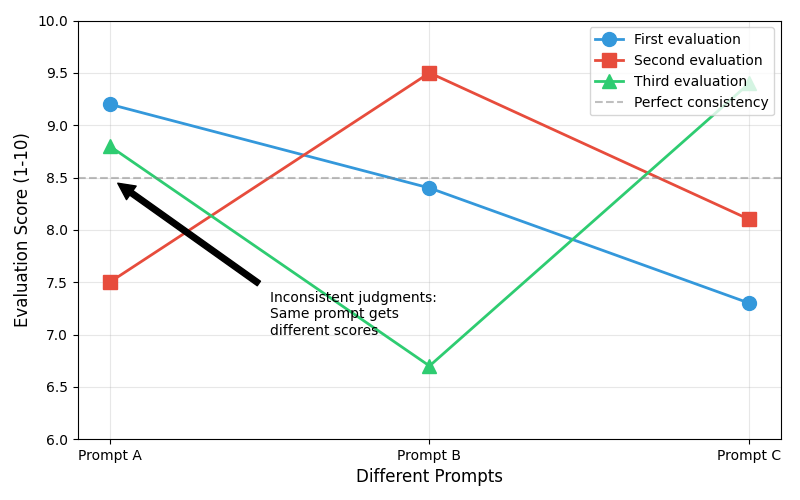
There are a few ways to address this. You can treat this like how we treated familiarity bias. Instead of prompting an LLM once, prompt it multiple times (5 times) and keep the majority output. But because this option can increase cost a lot, you can try to more intelligently prompt the judge itself. Try something like chain-of-thought reasoning, and ask the model for its reasoning before outputting a binary score. This can force a more deliberate consideration and reduce random variation.
We are an intermediate stage of LLM evaluation.
Current LLM judges are useful but imperfect tools. So, where does that leave us?
Start by identifying which biases most significantly impact your specific use case. For customer support bots, length bias might be your primary concern. For factual assessment, inconsistency may be more problematic. Apply targeted solutions for the problems you are immediately seeing, instead of trying to solve every issue at once.
We can expect evaluation techniques to continue to improve. Remember the goal is not to implement every possible mitigation, but to build a system that provides consistent and actionable feedback.
I hope this guide was helpful. Please comment if you have any questions.
Happy judging!